The original research in this video is made possible by generous contributions from supporters of the Dr.SHIVA Truth Freedom Health® movement. Please contribute so we may continue to bring you such original research, valuable education, and innovative solutions.
Key Points
- Dr.SHIVA Ayyadurai, MIT PhD – Inventor of Email, Systems Scientist, engineer, educator – presents a analysis of the ECHOMAIL® AUDIT OF BALLOT IMAGES & CAST VOTE RECORD (CVR) FROM 2020 U.S. PRESIDENTIAL RACE IN MARICOPA COUNTY, AZ
- Why was analysis of Ballot Images & CVR not performed as the first step in the Maricopa Audit? Millions of taxpayer dollars and time could have been saved, and such effort could have been directed to real issues, e.g., chain of custody, signature verification, to advance election systems integrity.
- EchoMail® – A.I. Ballot Image analysis identified 15,546 and 16,437 ballots that did not concur with Dominion A.I.’s pre-adjudicated tabulation and post-adjudicated tabulation, respectively. The Audit should have focused on these paper ballots versus reviewing all 2,089,563 ballots.
- Why are 6,835 Modified Cards missing? Per policy, for every Early Voting Ballot (EVB) where an Original Card in the Presidential Race was flagged for adjudication, there should be a Modified Card.
- When is a vote a vote given the inconsistent use of ‘Is Vote’ flag by the Dominion A.I. system? Even experienced election officials cannot explain the inconsistencies.
- Why are any ballots – even one – being adjudicated for the Presidential Race when the Original Card was never flagged for adjudication?
- The legislature, not a private corporation, must define the actual parameters that the A.I. should adhere to in determining a vote, write-in, undervote, overvote, or ambiguous.
Full Report:
EchoMail-ESII-Ballot-Images-Analysis-Maricopa-County-2BACKGROUND
Good evening everyone. It’s Dr.SHIVA Ayyadurai. I’ve been a little bit away from doing these broadcasts, particularly for the last two weeks. I try to do regular videos but I actually have to do a significant amount of work also, on a day-to-day basis, because some of you know, I run a number of businesses. And also, we’ve been heavily involved in wrapping up two reports that were commissioned for – by the Arizona Senate – on completing the audit of particular aspects of the Maricopa County Audit. Some of you may know, in 2020 – September of 2020, of this year (sorry, last – Jesus, is it? Are we already in 2022?) – I’m sorry – September of 2020, many of you may know I ran for office in the Republican primary and where we clearly saw that a landslide victory (which was due to us from all the hard work we did – 3000 volunteers on the ground – the immense work we did) was stolen by the work of the Massachusetts GOP.
And we discovered in – on September 9th of 2020 – that the election’s office in Massachusetts had deleted Ballot Images. And that’s what we’re going to talk about. And that started my journey (as someone – as a person who had great – who still has great love for this country – but wanted, as a scientist and an engineer, to run for office – and an Engineering Systems person) to recognize that the Election Systems in this country have serious, serious problems. Particularly, election officials can violate election laws by deleting what are called, “Ballot Images.” So, you’re going to learn what those are.
So, that started my journey between September of 2020 – to the present day – to really understand Election Systems and as we would understand any Engineering System. During the Maricopa Audit, I was called in to initially do Ballot Image analysis. And you’ll talk about why we were never given those Ballot Images, until after the Audit. So, that’s why the title of this said, “Why NOW?” were we doing this. But I did, in September of last year, while commissioned by the Arizona Senate to analyze the envelope images, where mail-in ballots are put in to look at the presence of signatures – and as I’ll talk about in the history – we persisted, even after that project, to try to get the Ballot Images.
And on December 7th, we were given the Ballot Images through a different source. And today you’re going to learn a lot about Ballot Images. What are Ballot Images? Why are they central when an audit is done? And the questions are why wasn’t this done first in the Maricopa audit. Why were we delayed in getting the images? Why were the images, essentially, when we got them, corrupted? Okay? So, there’s a lot of very interesting questions on that side.
But more importantly, I hope this serves as a way for policymakers to understand the importance of what’s going on in the field of artificial intelligence which is used in Election Voting Systems. Number two, for the public to really understand the scope of what goes on when you put a ballot in – and what goes on from the time the ballot goes in, to the tabulators, to how the vote is calculated.
And this is something, and frankly, it should be taught in civics’ class. And why it’s important to do that part of the audit. You’re also going to learn the various issues we discovered and what this means to all Americans. So, I hope this is helpful. So, without further ado, I’m going to start this very detailed presentation. So, you’re going to have to listen carefully because there’s a lot of stuff we’re going to be covering here. And I believe it’s going to be very valuable for everyone to have the opportunity to learn and study this.
ECHOMAIL® AUDIT OF BALLOT IMAGES & CAST VOTE RECORD (CVR) FROM 2020 U.S. PRESIDENTIAL RACE IN MARICOPA COUNTY, AZ
IF ARTIFICIAL INTELLIGENCE (A.I.) DRIVEN ELECTRONIC VOTING SYSTEMS CONTINUE TO BE USED, LEGISLATURES (NOT PRIVATE CORPORATIONS) MUST DEFINE THE PARAMETERS USED BY SUCH A.I. IN VOTE TABULATION
So, let me just jump right in. So, the title of this presentation is called, “EchoMail® Audit of Ballot Images & Cast Vote Record (CVR) from 2020 U.S. Presidential Race in Maricopa County, AZ.” The subtitle which really gets to one of the important points is, “If Artificial Intelligence (A.I.) Driven Electronic Voting Systems Continue To Be Used, Legislatures (Not Private Corporations) Must Define the Parameters Used By Such A.I. In Vote Tabulation.”
That’s one of the central aspects that emerges from the work we’ve done here. And it’s a very, very important understanding that you’re going to appreciate as I go through this. Okay? For those of you who don’t know me, my background is as an engineer, in electrical engineering and computer science, from MIT, in Visual Studies – and this is actually a master’s in visual studies out of the MIT Media Lab, a master’s from – in mechanical engineering where I did a lot of computational work, as well as in biological engineering – massive amounts of computing work on modeling things, like the whole cell. And this will be delivered to – in fact, we’ve gone over this with the Senate liaison, to Senator Karen Fann, as well as to Mark Brnovich, who’s the Attorney General of the state of Arizona.
ELECTION SYSTEMS INTEGRITY INSTITUTE
So, if you want to go look at this, there’s a link below in this, on YouTube or Facebook, where you can find the link to the presentation. It’s up. I just want to let everyone know, procedurally, EchoMail®, in affiliation with the Election Systems Integrity Institute, which is an institute that we launched about at the beginning of the year, is dedicated to providing independent research and infrastructure to support Election Systems Integrity. We take an Engineering Systems Approach at ESII. And ESII is really the publishing arm, which took a lot of this knowledge and puts it out there – so everyone can consume it. So, I hope everyone takes advantage of it. But EchoMail® was the one commissioned by the Arizona Senate to do it.
So, if you go through the report, I’m going to give a quick Executive Summary, some Foreword, a Summary, what we call the Abstract, the Project History, Introduction to what goes on. You’re going to understand what Ballot Images are, tabulators are, what CVR means. You’re going to also understand the Methodology we used, the results, and conclusions. So, lots of slides, but I go through them pretty quickly if you’ve been on any of my other videos. And then we’re going to also talk about Future Research that we’re planning on – what I consider the big piece of research – that actually should have been focused on.
EXECUTIVE SUMMARY
So, let me begin by, first of all, giving you an Executive Summary – if those of you who have to get to bed but want to know what the key points are. These are the key items for the Attorney General and the Senate to consider. First of all, I want to begin with asking this general question: Why was analysis of Ballot Images and Cast Vote Records not performed as the first step in the Maricopa Audit?
Millions of taxpayer dollars and time could have been saved, and such effort could have been directed to real issues – chain of custody, signature verification – to advance Election Systems Integrity. The EchoMail® AI Ballot Image Analysis work we did identified 15,546 and 16,437 ballots that did not concur with the Dominions AI, pre-adjudicated tabulation. What I mean by that is, when your ballot goes in, it gets converted to an image.
And artificial intelligence on these systems, first, looks at your votes, and then decides how they – if they – if they’re good, based on what the AI sets, or whether they should be reviewed by a human, which is called adjudication. Okay? So, we found 15,000 plus and 16,000 ballots that did not concur. And the reality is, with this kind of System, the Audit should have focused on those paper ballots versus reviewing all 2 million ballots.
So, imagine a surgeon just going in and doing surgery right away, as I was mentioning this to someone before the call, versus actually doing, you know, initial diagnostics and biopsy. It would be very, very wasteful to just go and start cutting up someone. You know? And this is why Ballot Images analyses are key. And I’m really, frankly, very perturbed, that the guys who led the Audit didn’t do this and they delayed. And you’ll see why. And that’s why I think the public needs to know about this.
So, the second bullet is we found that there were 6,835 Modified Cards – were missing. Modified Cards are when a ballot is adjudicated. For every ballot where there’s, let’s say an issue that the machine finds, they’re supposed to be adjudicated – except for in-person voting. But we found that 6,835 cards were missing. And per the policy, for every Early Voting Ballot, when an Original Card in the presidential race was flagged for adjudication, there should be a Modified Card.
The third big point is that when is a vote, a vote – because we’ve – given the inconsistent use of what we call the “IsVote?” That’s what they call it. The Dominion systems call it an “IsVote” flag by the Dominion AI. So, if a vote is filled in with a circle, for let’s say, Biden, then it flags it as an “IsVote.” And you would think that that is done. However, we found inconsistencies in this. And we even spoke to election officials with seasoned experience and they weren’t able to explain these inconsistencies.
The next point is why are any ballots, even one ballot, being adjudicated – which means going under human review – for the presidential race, when the Original Card was never flagged for adjudication? This is a very, very big issue. This is like, I would say, the equivalent of sort of the O-ring issue where people may pooh-pooh it saying, “Oh, what’s the big deal? It’s only one or two ballots.” But we found this and it took us a lot of effort to find this. We found a set of ballots which were never flagged by the AI for adjudication and somehow they get adjudicated later. It’s almost – matter gets created out of thin air.
And then finally, this is the central point that everyone should go with. And I think this is an issue that no one has really brought until we did it here. I have to emphasize, there’s three groups of people in the Election Integrity Movement. One is the people who deny there’s any problems. The second are the grifters – horrible people. They know that there’s a real problem. They don’t want to address the real problem. They’ve been making money off of it. And people have been funding these people.
And initially, I thought it was just incompetence, but I believe it’s more than incompetence. I believe it’s more nefarious and those grifters have been added to hide the real issues. This Audit in Maricopa should have begun with Ballot Image analysis. And it didn’t. And in fact, we were contracted for it and we weren’t given the images, as I’ll talk about. But the big issue that comes out of it is that the LEGISLATURE – not a private corporation – must define the actual parameters that the artificial intelligence should adhere to in determining a vote – write-in, undervote, overvote, or ambiguous.
The AI systems are the ones that are DETERMINING the process here. And I don’t believe most legislators even know what the rules are. It is the legislators who should be telling the AI how to do that. And this is a problem that we have when we have politicians who don’t understand technology. And that’s what we want to – we hope that today’s conversation – or the findings we have – the Audit we’ve done on the Ballot Images – will really help change policy. So, let me – So, that’s the Executive Summary. Okay.
FOREWORD
Foreword. Okay? This is what we have in the report. So, our analysis of Ballot Images and Cast Vote Records, in our view, should be the first step in an Engineering Systems Approach – because it provides a fast, cost-effective method to reveal discrepancies in vote counts, inconsistencies in adjudication, and questions with policy and compliance that election officials may want to address up-front. Right? You do this first.
Now, millions of dollars, as I said, were spent on the paper ballot counting (which revealed the numbers, you know, were pretty much the same) while self-serving grifters raised money for themselves and misdirected attention from real issues. And there are some major, major issues here that everyone should be concerned about. These are the real issues – chain of custody and signature verification – which another major report is forthcoming, from us.
Now, we completed this process – the whole thing that I’m sharing with you here – in 1 month. And we were supposed to be paid $50,000. And we’ve never been paid that. And the issue is why did EchoMail® have to struggle so hard to get the uncorrupted Ballot Images from Cyber Ninjas – the official Audit firm, who had the uncorrupted Ballot Images in their possession since April of 2021, and gave them to others, but not to EchoMail® – who was officially contracted to do the analysis. Why was that done?
And the final question is – was this process – why was this process the last step done for the audit. So, in the title of this presentation, I said, “Why NOW?” are we doing this? We wanted to do this a long time ago. It was AFTER the audit, which is so ironic, that we got the Ballot Images. Okay? So, anyway, I want to make people – these are the questions that come out in the Foreword.
ABSTRACT/SUMMARY
Let me continue. So, let’s cut to the Summary. Okay, the key things. First of all, the EchoMail® analysis of Ballot Images, as I mentioned, should have been the first step, not the last step. So, what is – why do I say this? This is the – this diagram represents the entire process – the real fundamental – the guts of the process that occurs when a paper ballot – after the envelope is opened. Okay?
So, we’re not talking about what happens in the envelope images – that’s a whole other thing before this. But, once the paper ballot is put through the tabulators, a Ballot Image is created, right here. Then, this is what I want people to understand in a very profound way. The machines have an artificial intelligence, of digital image processing, that literally analyzes this image and decides what the votes are. These are called pre-adjudicated.
And then, anything that it has doubts on which could be write-ins (are always considered adjudicated), undervotes, overvotes, and ambiguous. You’re going to understand what those are. Those get adjudicated by humans. And then after all of this, you get the CANVASS report, which is a final vote count. But just think about what I’m sharing with you here. This is a process. And the Ballot Image Analysis – long before you go, you know, spending millions, 10s of millions of dollars, a tremendous amount of time – should be what you want to do – the Ballot Image Analysis.
This is why I believe these election officials do not want to give these Ballot Images. But in Maricopa, the Ballot Images were made available and they were not made available to us – by the Audit organization who had them in their possession. So, why did they do this? Did they want to do paper ballots and do – raise a lot of money? Were they concerned that if we did the paper Ballot Images it would have shown everything was fine – that you would have had to focus on the non-grifter issues? This is a very important question that we must bring to the forefront.
But what we discovered – The EchoMail® Ballot Image and CVR Analysis – We completed this in 4 weeks. Let me remind everyone, this was done in 4 weeks by 2 people in 1 man-month. Okay? And we have yet to be paid. Okay. Now, what this revealed was that except for the disagreement on vote allocations of 15,000 ballots between EchoMail® and Dominion AI, vote allocation on the remaining 2,074,017 ballots concurred.
It also revealed that the Ballot Image Analysis would have directed, if we did it in the initial phases of the Audit, to review a finite set of paper ballots versus reviewing all of the 2,089,563. This would have saved time, money, and directed efforts to investigate discrepancies in vote counts, inconsistencies in adjudication, and questions with policy and compliance for election officials. We also identified significant inconsistencies in the manner in which ballots are adjudicated. And this really reveals a need for transparency in how these modern AI systems decide vote allocations.
And as I mentioned, we were commissioned to perform the Ballot Image Analysis in August of 2021. Cyber Ninjas had the bona fide Ballot Images since April of 2021. Yet, Cyber Ninjas never delivered those bona fide Ballot Images to EchoMail®. Question is, why?
PROJECT HISTORY
All right, so as I mentioned – the Project History – April of 2021 – Cyber Ninjas had the disk drives from the County containing the bona fide Ballot Images, as well as a disk image. So, this disk image, on the blue, was the entire Server System Image, but they also had – the most important thing is they had the disk drives with the bona fide Ballot Images. Okay?
So, the next – on June 5th of 2021, the Arizona State Senate contacted us to discuss commissioning EchoMail® for the analysis of the Dominion Ballot Images.
In July of 2021, a verbal agreement was reached, that we would be a subcontractor to Cyber Ninjas.
The last week of July, we actually got what’s called a Cast Vote Records which is the documentation on how votes were tabulated – in what’s called a JSON format.
And then on August 1st, 2021, within 48 hours, our team worked very fast, bringing them into a relational data structure. So, we were able to have a recreation of the entire election, and we requested to Cyber Ninjas for the Ballot Images.
On August 5th, a formal contract was executed between us and Cyber Ninjas for $50,000 to process Ballot Images.
On August 9th, Cyber Ninjas started, or uploaded, the Ballot Images for EchoMail® to begin processing. And within hours, we discovered 70% of the Ballot Images are corrupted and unusable, and immediately, we notified Cyber Ninjas. Their response was that these are “encrypted.” Well, we showed them they’re not encrypted – that was false. Then they acknowledged that the Ballot Images are corrupted. And then we informed Cyber Ninjas that we couldn’t do the project until we got bona fide Ballot Images.
From that day on – to August 19, Cyber Ninjas went silent on the matter and never, frankly, made even the first payment.
On August 19th, we terminated that agreement and informed the Arizona Senate Liaison of the “corrupted” Ballot Images. And Cyber Ninjas went public and blamed the County for corrupting the Ballot Images. Okay? When they actually had, in their possession, the actual uncorrupted Ballot Images.
On September 1st, 2021, we were commissioned by the Arizona State Senate for signature presence detection on a new project, on the envelope images.
And on the 24th of September 2021, we presented that information, but we also exposed various anomalies and raised new questions on signature verification.
On the 19th through the 23rd – November 23rd, of 2021, we persisted to find out where the uncorrupted Ballot Images were.
On November 23rd, 2021, Randy Pullen, the Arizona State Liaison, was able to locate the bona fide Ballot Images from Ben Cotton and arrangements were made for us to get it.
Interestingly enough, on November 23rd, 2021 , I actually met with President Trump in Mar-A-Lago and informed him that Cyber Ninjas never gave us the Ballot Images. And the interesting thing was President Trump asked, “What are Ballot Images?” So, I had to explain that to him. So, here’s the potential victim of this situation and he didn’t even know what Ballot Images were – nor had anyone informed him about it until I met with him on November 23rd.
So, in early December, 2021, Ben Cotton, one of the people who participated in the audit, informed us that Cyber Ninjas always had the uncorrupted Ballot Images in their possession. And Cotton revealed that Cyber Ninjas had indeed sent uncorrupted Ballot Images to other parties, back in June. So, he was confused why we didn’t get it.
On December 7th, we finally received the uncorrupted Ballot Images from Ben Cotton, after the official, after the official Audit by Cyber Ninjas had been completed. In testimony to the Arizona State Senate, Cyber Ninjas alleged that the Ballot Images were corrupted by the County. They had them in their possession.
So, on January 7th, within 4 weeks of getting it – we completed the Ballot Image and CVR Analysis and presented the findings to the Arizona State Liaison.
On January 22nd of this year. I’m sorry, this is wrong, I need to edit it. Mistake right here. I’m going to do it right now. Error right here – this should be January 22nd, 2022. We’ll make that edit also on our slides. So, I’m just going to go back to this. So, there’s an error there that I just made – I got the year wrong – because we’re in 2022. So, let me do that again. I hate doing that. So, let me go back to this. So, that is the timeline and I think it’s important for everyone to understand what took place. Okay, because this is a – the project has a history.
So, January 7th, 2022, we finished the analysis. And here we are today where we’re presenting this to you. So, that’s the whole project history.
INTRODUCTION
So, let me give you a little bit of background for those of you who are new to this. Congress actually passed a law, in fact, by a democrat majority about 50 years ago, to encourage audits. Audits, if you said you want to do an audit, no one should brand you as unpatriotic, or you’re trying to hurt the Election Systems. In fact, it’s a good thing. So, pursuant to 52 USC 20701 – that’s the law – it encourages citizens to conduct election audits – all records, in fact, per that law, that are generated in connection with a federal election are supposed to be preserved. Right,
“All records generated” include Ballot Images and Cast Vote Records. Ballot Images include the pre, which means the version done by the machine automatically, and post-adjudicated. So, think about what happens. A ballot comes in, right? It needs to be – it goes in, it gets imaged, and then the machine is counting the votes. But if the machine, through its AI, cannot determine what’s right, then it gets sent for human review. And that’s called – and after they review it – that’s called post-adjudicated.
Okay. Now, the Election Systems Integrity Institute, in collaboration with EchoMail®, is publishing the results of the Ballot Image and CVR Analysis. That’s what we’re doing tonight – of the 2,089,563 Ballot Images.
All right. Now, this report documents the analysis we did, which was completed on January 7th.
Now, in precincts and counties using electronic voting systems, the AI is used to analyze those images to tabulate the votes prior to adjudication. Okay, so what happens here is the machine looks at – looked at all those 2,089,000 plus ballots and figured out vote counts. Okay. The ones that it didn’t – couldn’t figure out – or it was unsure about – those were put into a pile called “adjudicated” which are reviewed by humans. Okay. But it’s key to understand that the AI is doing this. Okay?
Now, before Electronic Voting Systems, election officials used humans, right, to review a ballot and determine vote counts, as well as what ballots need adjudication. So, think about before when we were doing hand counts. A human was told, okay, if you see it filled in all the way, that oval, that’s a vote. If you see that filled in and another one filled in, that’s called an overvote.
If you see none of the ovals filled in, that’s called an undervote. Okay. If you see some stray marks – they were given what rules to do. Suppose one oval, let’s say for Trump, was filled in 50%, and for Biden, maybe it’s filled in with just a little dot? Well, depending on what rules the human was told, they say, okay, that’s a vote for Trump. The AI is now applying its algorithms. Does anyone know what those algorithms are? Where is that dictated? And that’s what the heart of our investigation discovered. It’s a very, very important issue. Okay?
So, adjudication is a process where more than one individual, likely one from each party, reviews a questionable ballot to determine the vote allocation.
Now, clear guidelines were established when humans reviewed a ballot, as I just mentioned, if it was bona fide, undervote, overvote, etc. Now, any undervote, overvote, write-in, and ambiguous vote was always set for human review. Okay, for in-person voting, in some counties, as in Maricopa, the adjudication is done by the voter for in-person. So, if you go in-person and the machine says, hey, did you mean both of these – or this? The human being does it, so those do not go for human adjudication. Okay, so we’re talking about the mail-in, early voting ballots.
Now, such guidelines – for example, when you told the human – were, how much of the oval was filled in to be a vote? What were stray marks?
Now, in the modern world of electronic voting systems – with essentially – you have “robots” doing this, right – you have the robots literally looking at the – or the AI – to determine votes, undervotes, overvotes, write-in, and ambiguous. So, now it becomes important to know what are the standards being used by those robots, by these private companies who programmed their machinery. When is a vote a vote? What percentage of the circle or oval needs to be filled in? When is a vote determined by the AI to be an undervote? Suppose there are some minor marks. When is a vote an overvote? Suppose one oval is filled in 95% and the others have 5% filled in.
So, bottom-line, there’s a need for transparent standards of how AI, on such electronic voting systems, classify votes. By the way, if such standards exist, this should be made easily accessible to the public without the need to do public records’ requests. The public should be made fully aware of how their votes will be tabulated by these AI.
Now, in my view, this is going to serve a two-fold purpose. First, if people want to keep these electronic voting systems, it’ll increase people’s trust. But if the AI is selecting and we don’t know, that’s a serious issue why people want to get rid of the machines. The second is, it’s going to enable independent audits, like what we just did, to occur faster, better, and more cost effectively. So, I hope that – that’s an Introduction now. Okay. I hope that helps everyone understand the foundational elements. Okay? So, if you want to take a glass of water or something, let’s go into the next part of this presentation. Okay.
BALLOT IMAGES
Again, it’s a very – the goal here is to be educational. A lot of this is new. I’m consolidating about 2 years’ worth of knowledge in the next, you know, 90 minutes here. So, what is a Ballot Image? This is when a voter submits a paper ballot, mail-in, or in-person, and the ballots are processed geographically. In the case of Maricopa, there were centralized tabulators and scanners at what’s called, MTech, MCTEC, for early voting. I think it’s Maricopa Commission on Election Commission – I forget the exact name – for Early Voting Ballots. Right. So, all the Early Voting Ballots were centrally tabulated and scanned. All the other ballots were decentralized using tabulators across Maricopa for in-person ballots.
So, for example, there were 2,089,563 ballots. And guess what? There were 2,089,563 Ballot Images. The advantage is these are digital formats. Right? We could process these super-fast, if we got the Ballot Images. And we did that in 4 weeks – very quickly. We believe at EchoMail® we can do this in hours. Right? So, we can do rapid audits very, very quickly. We have the capability to do that, if people want us to do that.
Now, the tabulators are used to process those paper ballots, as I mentioned, to generate those Ballot Images.
In Maricopa, 2,089,563 Ballot Images were generated, representing all the ballots cast that were generated from 379 tabulators/scanners in Maricopa.
Now, the 1,923,719 Early Voting Ballots were generated – those generated images from 33 of the centralized, MCTEC centralized tabulators.
The In-Person Ballot Images were from 165,844 In-Person votes. And that was produced by – look, a lot more – 10 times more tabulators because they were spread across all the different precincts. So, basically, if you voted by mail or early voting, those went to a centralized set of tabulators. And you’re going to see how the images were very different – looking – in fact. And if you went to vote in-person, even though it was less votes, it was spread across 300 plus different scanners. Okay? All right.
CAST VOTE RECORDS (CVR)
What are Cast Vote Records? Okay, you may have heard of these. But again, you’re going to learn here – Cast Vote Records, also known as CVR. So, Cast Vote Records are the repository for documenting the vote allocations. They contain in them something called the “Original Card.” An Original Card is when the AI – when the AI machine went through and processed those Ballot Images. It creates what’s called, in the CVR, an Original Card for every race, saying, hey, this is what it thinks the votes are. Okay?
Then, if anything gets adjudicated, it flags it for adjudication. Then, there’s a process where for every Original Card where there’s an adjudication, a Modified Card exists. Okay? But this is important to understand. You may have multiple races on a ballot. It’s not just only one race that may require adjudication, but only one Modified Card gets created for one Original Card, whether there’s 1 or 100 adjudications flagged. Very important to understand. All right. And then the Deltas – you know – how things change. So, the CVR is extremely important because the Cast Vote Record file literally tracks the atomic journey of a ballot. You know, how it went, how it was flagged by the AI, how it was adjudicated, etc. So, that’s the process this goes through.
And the CVR is used to tabulate the final vote count. So, in the CANVASS Report – so, when the final vote count is accomplished – CNN says this is the vote count – that’s coming from the post-adjudicated CVRs. Okay?
BALLOT IMAGES & CVR
All right. I’m going to go through this, you know, in a couple of different ways, so you’re all going to get it. Okay. So, now, how do the Ballot Images in the CVR work? Well, the AI on the tabulators performs the image analysis. Right?
The AI determines the vote counts on each race (or question).
The vote counts are stored in the CVR as what’s called an “Original Card” – the pre-adjudicated state.
Then the AI flags races on the Original Card for “adjudication” – undervote, overvote, ambiguous and write-in. These are what are flagged for adjudication. Write-ins are always adjudicated by humans.
“Modified Cards” are created for ballots flagged for adjudication. Every flagged race on an Original Card must have a Modified Card. The exception, like in Maricopa, is for in-person voting, where no Modified Card is generated. So, just keep that in the back of your mind.
METHODOLOGY
So, what did we do? So, now you got all the background and the introduction, the project history, how we got here, and some of the reasons we were very frustrated were that we never got the Ballot Images. Now, we’re going to talk about what we did. So, on December 7th, we got the Ballot Images, finally. And thank you to those people who are donating to us. It’s again, you know, this project we did on our own – millions of dollars were spent on the Audit.
The most we got was for the envelope images for $50k. And we did probably work on the order, frankly, without hyperbole, probably 100 times that value. But we did this because we’re very concerned about what’s going on in this country. So, let me go over here. What did we do – The Methodology. The Methodology we used is first we acquired all the Ballot Images and the CVR. We got the CVR back but we never got the Ballot Images.
Then we organized all those Ballot Images and CVR into a relational data warehouse. What does that mean? We sucked in all that data and put it in, in our data center, into a relational data warehouse so we could identify things very, very quickly. Okay?
All right, next thing we did was we executed – many of you know, my specialty for nearly three – since – since I was a 14-year-old kid, in 1978, has been pattern recognition. I built some of the first systems to analyze sleep wave patterns and ultrasonic signals was my master’s work. And my PhD work was in looking at computing but for all different kinds of image analysis and handwriting recognition – all different kinds of stuff. It’s an area where today you call it AI, before it was called pattern recognition. So, we have created, at EchoMail®, a technology for that. We, it so happened that we started a company to use it for email, but we can use it for anything. We’ve, in fact, used it for other things. So here, we applied that same pattern recognition methodology to look at the Ballot Images to tabulate results.
So, we looked at, to be specific, we looked at the presidential race, we looked at, you know, if a dot was for Biden, or Trump, or a third-party candidate, known as Jorgensen, or if was a write-in. So, we did all that analysis. Hey, John, can you get me some water? Thanks. So, we did all of that analysis. Our AI ran through 2,089,083 images. And our system can do about, you know, in .4 seconds, we can analyze an image. And so, we processed all of those images and we calculated our vote counts. Okay? What would our AI have gotten? And I’ll share those results, okay.
But in addition to that, we then performed discrepancy analysis and we did four phases of analysis. First, we looked at the post- adjudicated – so we started backwards and I’ll show you how we did this. We looked at what the County reported as their votes and what was in the post-adjudicated CVR. Then, we looked at the pre-adjudicated CVR, which means what the machine predicted, the Dominion AI, and how it was post-adjudicated. And then we compared our AI with the Dominion AI analysis. And then we compare our AI with the CANVASS Report.
And then we itemized issues and concerns. Okay. So again, let’s look at this diagram here, for those of you who are joining. This is Dr.SHIVA Ayyadurai and we’re sharing with people, hot off the press, our Audit of the Ballot Images from the Maricopa Audit for the presidential race of 2020. And it’s being done now because we only got the Ballot Images on December 7th.
Okay, so let’s go over here. And what you see here is this is a System. Paper ballot comes in. The tabulators create a Ballot Image. The machine, in this case, the Dominion machine, used its artificial intelligence to figure out what those votes were, and put it – and these are called pre-adjudicated in the Cast Vote Records – anything it thought it was an overvote, an undervote, a write-in, or ambiguous, got sent to a human. And those humans reviewed those ballots and they were adjudicated.
And then you got the final CANVASS Report. So, the first thing we’re going to walk you through is, we looked at what was reported and what was in the CVR. This was just a mental check. Do these make sense? They should be perfect. Okay, so we did that. Then, we went back and we said, Phase II. How were the pre-adjudicated, which means what the machine said, and the post-adjudicated state? How did that all of that occur? And this is very, very complicated. Okay? We did that analysis and found various policy issues. We, in fact, spoke to county officials in Arizona. And they weren’t even – and these are very experienced people – and they weren’t even able to answer these inconsistencies.
The reason we did that is anytime we put stuff out there and we ask questions, the election officials in the County say oh, Dr.SHIVA didn’t know this, didn’t know this. Well, to the county officials listening, and to the Attorney General’s office in Arizona, and to the Senate, we are more than open, and welcome and invite the County to have discussions with us, in particular on these inconsistencies we found. So, the second part we’re going to walk through is a Phase II where we look at the pre-adjudicate and the post-adjudicated. Then, what we did was EchoMail® analyzed the images and got numbers – our AI. And we compared our AI to the Dominion AI. And we’ll talk about that. And then, finally, we compared our AI to the end report. Okay.
RESULTS – PHASE 1
So, let’s go right to the Results. So, the Phase I Results are looking at our CVR analysis, which means looking at the Cast Vote Records, post-adjudicated, and the CANVASS Report. Now, mind you, this should be perfect. Okay. And they were. Okay? So, that’s good news. Okay? Meaning, good news, meaning that what the machine had stored matched what the County had reported. So, that’s Phase I.
PHASE 1 – Summary
So, Summary here is the CVR analysis of the post-adjudicated state reveals that the Maricopa CANVASS Report concurs with the post-adjudicated CVR, and there’s no variance – Phase I.
RESULTS – PHASE II – PART 1
Let’s go to Phase II. And we have various parts in Phase II. So, Part 1 was we now wanted to determine how many ballots were flagged for adjudication that included the presidential race. Again, so what we did was we audited. We went through the CVR, which is a very complex file structure, and we calculated, from the CVR for the Maricopa Audit, how many of those were flagged for adjudication, which means that the machine said that it was undervote overvote, ambiguous or write-in.
Okay, those are the three categories that says a human being should look at it. And this is what we saw. Out of the 2,089,563 votes cast, 2,063,866 were Original Cards, which means the machine on the first shot got votes for Trump, Biden, or Jorgensen. Okay? This means these should never be adjudicated and listen to this very carefully. Okay. It means these votes were tagged with a flag called “IsVote.” Okay. What we were told was a unique vote. Okay, which means the machine said definitively for Trump, definitively for Biden, definitively for the third-party candidate, Jorgensen.
So, that’s what we see here. However, the machine flagged for the presidential race – we didn’t look at all the races – 25,697 ballots, where it needed to be adjudicated, which means reviewed by humans. Okay. And by the way, we have included in an Appendix, all those ballot images for the Attorney General to review Okay, so we, again, it’s gigabytes worth of data that we’ve isolated. So, these are those 25 – So, according to the Dominion Systems, 25,697 were flagged for adjudication, based on our analysis of the CVR. Okay, so there you go.
PHASE 1I – PART 1 – Summary
So, what did this part summarize – Phase 2, Part 1. So, the CVR analysis of the pre-adjudicated reveals 25,687 were flagged.
Now, “Flagged for Adjudication” means those ballots, again that the Dominion determined were write-ins, overvotes, undervotes, and/or ambiguous. Write-ins, you know, are write-ins. Overvotes are if someone voted for more than one person, like Trump and Biden they circled. Undervotes are – they didn’t fill in anything. Ambiguous could be there was a stray mark on it. Okay? Someone spilled coffee, you know?
Now it’s important to note the ballots, right, that a ballot, the Original Card, may be flagged for multiple reasons. By the way, on one run race, someone may have written in, and maybe didn’t fill, didn’t fill any of the circles out. Or they wrote in and they didn’t fill anything, and there was an ambiguous stray mark. So, multiple things could be there.
RESULTS – PHASE II – PART 2
All right. Now let me go to Phase II, Part 2 here. This was a very, very deep analysis. Now we’re going into the bowels of the CVR. And we’re going through with minutia and we’re trying to understand how were – what were these flagged adjudications. Right? So, 25,697 ballots were flagged for human review for the presidential race. Now, a couple of points here that I want to mention. For early voting ballots, like mail-in ballots, if something is flagged for adjudication, you have the original ballot, what’s called the Original Card stored.
But if it’s flagged for adjudication, again, only for early voting ballots, then a Modified Card gets created, which means that’s for the human reviewers to review and update. Right? They make modifications. Even if it said undervote here and it’s undervote, it’s still a Modified Card. Again, that’s only for early voting ballots that were flagged for adjudication. In Maricopa, for in-person votes that were done – let’s say you put it in the machine and the machine said hey, you voted for two people – the adjudication is done right then and there, as we’ve been told, and no modified card should be produced. So again, from the policy perspective, Original Card – a Modified Card – should always be produced for every Original Card/ballot, where it was flagged for adjudication, in the case of an early voting ballot. Okay?
I know I’m hitting you with a lot, but it’s a learning opportunity for everyone. So, that’s what should happen, but what did we find? Let’s go through this. So, what we find is, first of all, flagged Original Cards, which means ballots, with Modified Cards with changes. That was 18,402. Okay? Of these, 17,344 were early voting ballots. But here’s something interesting. There are 1,058 in-person ballots. According to their policy, you should not have a Modified Card for these, right, because the Modified Cards are only for EVBs. So, you find these Modified Cards?
Again, it’s a policy question. This is not something about it’s going to change, you know, the election – the results here. But this is a point, they’re not following a policy issue here. Then, we found out – this is what’s a little more disconcerting. There were ballots flagged for adjudication, but they don’t have a Modified Card. And those were 7,295. And initially, we said, wow, that doesn’t make sense. And people said, no, no, no, you have to make sure, well, those all could have been in-person ballots. And we said, oh, maybe we made a mistake. But guess what we found when we did our analysis? Only 460 of these were in-person. 6,835 should have a Modified Card. And these don’t. And this gets even more interesting.
We found 11 ballots, and this is what I call the O-ring phenomenon. We found 11 ballots that had Modified Cards, but they were never flagged – they were never flagged for adjudication. So, these appear as Modified Cards, literally out of thin air. And this is more disconcerting. Okay, so when you look at it, there were 25,697 Original Cards. You should have 18,413 Modified Cards, because you should not have Modified Cards for the, you know, the ones that were – the – Well, I’m sorry, you have – actual was you have 18,413 Modified Cards, but we were expecting 24,179 Modified Cards. So, we’re missing, you know, 6,835 Modified Cards. So that’s the point that we want to make here. That in the adjudication process, it appears that county’s not following their own rules on how they track this. Okay. There we go.
PHASE 1I – PART 2 – Summary
Next, to summarize, again. I like repeating here because we’re making a lot of points. You know, out of the 25,697 ballots that were flagged, per the policy, right, you should have this many that were sent for adjudication. There should be – were sent for adjudication – however, only 17,334 Modified Cards actually exist. And a total of 6,835, 6,835 cards are missing, as I mentioned. Okay, now of these, 25,697, which are all of these here, 1,518 were in-person voting, and per the policy, should not have Modified Cards, but they do. Okay? However, there are only 18,413 Modified Cards for the presidential race. We’re missing Modified Cards. And moreover, there were 1,058 Modified Cards for in-person voting that were flagged for adjudication. Okay. Per policy, these Modified Cards should not exist.
All right. Now, I’m going to talk to you about something that I’m teeing up, that comes to a very, very big issue here. Okay? It’s that we discovered a potential inconsistency in the policy on EVBs flagged for adjudication. What do I mean by this? There were 11 electronic early voting ballots that were found to have Modified Cards. However, there was no Original Card, as I mentioned. And these 11 EVBs – we’re going to explore the research. Okay, we’re going to explore it later.
I want to emphasize that the amount of research that was required to decipher this was not small. I mean, literally, we had three PhDs, including myself. And that’s what I find a little bit disconcerting. That the complexity of this – it shouldn’t be this complex. Okay?
So, let me go now – Oh, by the way, all of these we put into a file for the Attorney General to look at. All right.
RESULTS – PHASE II – PART 3
Let’s go to Part III. The next thing we did was, and this gets – This is a lot of fun because we actually did a visualization through some Venn diagrams to actually – for people to understand, out of those 25,697 ballots, which needed to be adjudicated, how were they adjudicated. Remember, 25,697 ballots needed to be adjudicated, but on any one ballot, you could have multiple potential modifications. It’s not one to one. So, in fact, what we found was that of the 25,697 ballots flagged for adjudication, among those, there were 28,645 total potential modifications. Okay.
So, let’s begin with the process. So, ballots come in. They’re imaged. The Artificial Intelligence System, of Dominion, looked at all of those ballots. And it decided – it looks at those ovals, and it says, okay, this is definitely for Trump. How is it deciding that? Does the oval have to be filled in 95%, 20%, 30%? It’s – I think it’s important legislators know what that number is. But regardless, when the Dominion AI did that, what you find is this. Okay?
And by the way, when it flags something, it’s called “IsVote.” That’s not our terminology. That’s in the CVR. Again, the flag, IsVote, in the JSON database, means that this ballot IsVote for this person.
So, in this case, what you see here is that 993,973, in the first pass of the AI, were pure Trump votes, which means they should not ever be adjudicated. They’re done for Trump. They’re in for Trump. Nothing should ever happen after that. 1,038,321 for Biden. 31,572 were for Jorgensen. So, these are the allocations that were done by the AI. And these are only – they ONLY have IsVote vote flags on them. They didn’t have any other flags, meaning they weren’t flagged for adjudication at all. Okay.
Out of the 25,697, is that right? 25,697 – there were 931 ballots that were undervotes. Right? There we go. Which means people, people didn’t fill out any of the circles. There were 9,440, which were write-ins, and notice 9,421 were pure write-ins, or write-ins alone, but 19 were undervotes and write-ins, Then we had overvotes, which were 7,332. 5,877 were pure overvotes, which means they filled in two, but 1,455 were write-ins and overvotes.
Okay, that means they wrote something in but they checked off the write-in, and maybe they also, or filled the oval for the write-in and filled maybe for Biden. And then they were ambiguous. So, you notice. So, when you put all this together, there were 568 pure ambiguous. But among those 9,831undervotes, some of them were pure undervotes, just like some of them were pure write-ins, and some of them pure overvotes, but some of them overlap. These overlapping areas are why you get more potential modifications than the ballots that were flagged, All right.
Let me take a break. So, people sort of can reflect. So, what we did here was we actually understood how the ballots, when they came in, were decided by the AI. So, remember, no humans are involved here. The AI determined all of these allocations. Okay? And by the way, we tried to do this in a spreadsheet, and it got way too complicated for any human to understand. So, we did it in this way so you can understand this. Okay?
Now, what’s interesting is, if you look at it. I mean, there’s something interesting – you look at this 19. And one of the election officials we spoke to said, you know, that’s interesting – these look like the 19 that were flagged as undervote, which means, and write-in and ambiguous – so, these must have been voters that didn’t fill in any of the circle, or the oval, even the oval next to the write-in. But they just wrote someone’s name in. She was a little bit concerned there weren’t more of these. But that’s what – see – with this diagram, you can easily see these kinds of modifications, potential modifications.
What’s here that was interesting – I want everyone to listen to this carefully. And this gets into one of the major policy issues that are big, you know, significant issues that we think should be addressed by the legislators and particularly. There were – so here were pure Trump votes, but what we found was there were 191 votes, which were flagged for Trump IsVote. We were under the understanding IsVote means IsVote. You can’t have IsVote and also flagged for adjudication. It didn’t make any sense. How could you say, Trump got a vote AND it should be looked at for some other reasons?
Well, then you shouldn’t have it as IsVote. And this was something we found – and this took us a lot of effort to find this – is that there were things that were flagged as a vote for Trump but were also flagged for adjudication. This should be a one or a zero, IsVote, or this. And again, this is not clear. Similarly, 400 votes for Trump – uh, for Biden – which were IsVote, but also in one of these pools, and the same Jorgensen. And we’ll get to this.
So, the net of it was – that you’ll see here – that out of those total number of votes that were pure votes – you know, these were the pure votes, no adjudication – among the votes that were flagged for adjudication, you can see the write-ins, undervotes, overvotes, unambiguous. Okay. That’s what this diagram is really sharing. It’s just a summary. Okay.
So, what we did here was, if you consider putting these together, right, because these were also IsVotes. Trump, at a certain state in the CVR had 994,164, Biden had this many, and Jorgensen had this. These are pre-adjudication. All right. And that’s what we just shared with you.
PHASE 1I – PART 3 – Summary
So, the clear observations here that we want to talk about were, that this visual representation that we just shared with you here, provides a nice detailed understanding. Okay.
Now, in this analysis, we discovered 608 Original Cards that were flagged as IsVote for a candidate and for adjudication. So those are these here, this adds up to 608.
Okay, and again, this is a question: why can it be in IsVote and an adjudication. So, an important policy question arises: What does IsVote mean? And is it, is it a vote or not? If not a clean vote, then why is it flagged as IsVote?
And then EchoMail®, prior to publishing this document, did have conversation with two highly experienced election officials in Arizona. And they were unable to provide answers to this question, which I found very interesting. That we showed them this and we said, why is this an IsVote and this, and they said wow, we don’t even know. Okay, and these people have been working for decades in this area.
So, we invite a conversation with the county officials to have a discussion on the policy concerning the use of what is a vote. So, the question is what is a vote. Okay. All right, simple question, but the AI – and this is why the policy needs to be set.
Now let’s go to Phase II, Part 3. And I want to show you some examples. So, these are some examples of a ballot that is a vote. Right, but it’s also tagged as ambiguous. So, you can see it’s clear it was him, but there’s a little mark over here. Why isn’t it just a vote for Trump? Why was it also flagged as ambiguous. And if it was, then it should just be ambiguous. It shouldn’t be Trump got a vote. It should just be ambiguous. Similarly, here, you can see someone filled in Trump, but then they scratched all these out. This should have just gone to adjudication. It should not be an IsVote.
Same here in another case where you see that if this is not, you know, there’s a little thing here for Jorgensen and a big one for Trump. So, the real question comes in. How is the AI deciding what is a vote? What is the percentage that it’s got to be filled in? And that’s what we asked here. What are the density settings on how much an oval is filled, in comparison to another? Now, this is important because in the old days of mail-in or hand counted ballots, you had procedures, you told people. Okay? Now, we’ve outsourced that to a private company, who is deciding these very little minutiae.
Where is the legislation and the policy for this? Is it written anywhere that how the machine can decide this? So, in this case, two are X’s, and this was still given an IsVote for Trump, but not to Jorgensen, and ambiguous. It should not be IsVote because it is not an IsVote. It’s ambiguous because in adjudication, they figure it out. And here’s another example where it’s an X on Biden in a circle. And it also is put as an IsVote and ambiguous. Again, how – what are the criteria that the machine is deciding to do this? So, here’s also some examples on Biden. Okay?
Same thing – you can see this circled and this, and this is also a vote for Biden, but ambiguous. And same here. The real question here is, it should be either it’s adjudicated, but should definitely not be an IsVote. And so, this is again, you see a big line going through here. And again, this is also a Biden vote and ambiguous. And same here, this circle is sort of weirded out on Trump. And here, this also becomes ambiguous, but is also IsVote for Biden. And same here. And same here. Again, we ask these questions.
These are two X marks. And then, same thing with Jorgensen. You see the same examples, two marked. That’s an IsVote and ambiguous. Another example, it’s marked here and here. And again, the questions we are – what are the density settings that the AI is using? What are the conditions when it is an overvote versus ambiguous? And then, finally, an example here, and here – same case. And also, here, and here. Okay? And another case for Jorgensen up here, and here, where two are marked. All right.
So, the net of this Phase II, Part 3, of this analysis is that, you know, the important observation here is that the actual parameters that the AI uses in determining a vote, write-in, undervote, overvote, or ambiguous, should be fully transparent and dictated by the legislature.
So, to those of you who are lawyers, who have been involved in this, think about what we’ve discovered here, or uncovered here. That the machine is making all these pre-adjudicated decisions. And maybe it’s doing it accurately. Maybe it’s doing it perfectly. However, do we, as a public, have visibility on that? An AI machine is running – where is the standard on exactly what that is? If it’s there, it should be made public because maybe it’ll help people actually vote better. Maybe they wouldn’t be doing these X’s and all this other stuff. But it is a little bit disconcerting, not only a little bit, that the legislature should be dictating this to the AI. And it should be perfectly written up. That makes sense. Okay?
So, again, we have put all of these in an Appendix to be reviewed by the Attorney General. And again, as I mentioned, even if the AI is correct, it’s not prudent to simply trust that the AI will be accurate, without the public knowing what actual parameters the AI is using to determine vote allocations.
RESULTS – PHASE II – PART 4
All right. Next part is – now, this gets a little bit interesting because now we’re going to look at the pre-adjudicated state, what we just showed you. And then the post-adjudicated state. So, we went from the pre-adjudicated, what the machine did, to what the humans did. And when it went from here to here, what were all those atomic changes that took place? And can we trust them? Okay? Again, this was a lot of intense work we did in those 4 weeks. So, let me share that with you. All right, but this is what we call a serious Audit. This was done in 4 weeks, by 2 people. Okay? For $50,000, that hasn’t been paid. Okay?
So, here we go. So pre-adjudicated state. Okay. So, this is what I shared with you. These were the votes that were allocated to Trump except this triangle here, where they’re sort of, here and in this group. Right? They’re in purgatory. These ones here. But it’s the pre-adjudicated state.
After adjudication, which means human beings looked at them, and they said, okay, we decided these votes go here, here, and here. This is called the post-adjudicated state. Okay? How do they end up? Okay? We’re going to show you here, again, visually, because it gets too complicated. This is how they end up. So, what you see here is of these 994,164 votes, 995,665 Trump gets. Biden gets, out of the 1,038,721, 1,040,774. And this was reported in the final reports. And out of the 31,589, pre-adjudicated, Jorgensen got 31,705. Okay?
Now, when we look at the post-adjudicated, the write-ins, 7,942. And guess what? There were 7,942 Original and Modified Cards. So, we were fine with that, the EchoMail® team was.
When we did the overvotes, there were 5,002 Original and Modified Pairs. We were fine with that.
However, when we looked at the undervotes, we only find 1,180 Modified Cards. Okay? Although, there were 8,475 undervotes, and we’ll explain this. We expected more than this number of Modified Cards.
So, our analysis of the pre-adjudicated to post – it revealed that the write-ins and overvotes appeared to have been accurately calculated.
However, for undervotes, there was an inconsistency. Only 1,180 Modified Cards were observed in the post adjudicated state although there were 8,475 total post-adjudicated undervotes.
To resolve this required a significant deciphering effort across these two states. This was a lot of effort. So, let me walk you through this.
So, what we found was there was actually, of the 1,180 Modified Cards, 1,170 had Original Cards, which was fine. But this was what I was talking about, there was 10 Modified Cards, votes, that just show up and they don’t have any origin where the Original Cards were flagged. So, this again, goes back to that issue. Here we are finding 10 adjudications taking place, but they were never flagged for adjudication. So, votes get changed here – but you can’t – they weren’t flagged for adjudication. This is very disconcerting, which means how could someone suddenly decide to change votes over here, adjudicate them, when they were never flagged? Okay, major policy issue. So again, we asked these election officials, and they weren’t able to explain it. So, in this deciphering effort, EchoMail® discovered that the 10 Modified Cards had no Original Cards.
In fact, the Original Cards associated with these 10, were marked as IsVotes, pure IsVotes. So, they came from this pool, not even from the triangle. So, think about what I’m saying. There were votes that were absolutely for Trump, absolutely for Biden. No flags, but suddenly they get switched. Okay? All right. So, how did all this happen?
So, how did this happen? Again, this was a serious journey of a lot of sleepless nights. How did this happen? Well, in order to do this, we wanted to go back a state. So, if you notice, 995,665 was here for Trump – 1,040,774. So, we found there were 4 votes for Trump, right, that he had, and 6 votes Biden had. So, 6 votes Biden had went over here, and four votes. So, we went back and found that. We had to, essentially – it’s sort of like playing back a movie in time. So, we went back and we played back the movie.
And we said, okay, there were these number of votes that were in the, sort of pre-adjudicated state, and we followed this. What ended up happening actually was, for some, so for some reason, five of Trump’s pure votes, which were totally flagged from nothing flagged in the CVR – so, nothing was flagged in the CVR. This is important. That’s why the Ballot Images are important. In the CVR, if you looked at it, it’s not even flagged. But, these five suddenly have Modified Cards and they become undervotes. So, five votes that were purely tagged for Trump become then undervotes.
And what are those look like? Here they are. Okay, here were those five. So, they were flagged for Trump and someone put an X in it. They were never flagged for adjudication. How did these votes suddenly get tagged as undervotes? Okay? They weren’t done – the machine didn’t calculate this. How did a human being know about this later? I mean, did they – so, one of the election officials we spoke to, she said, well, maybe when they’re putting it in, they saw it. And the issue is, that’s quite arbitrary. How many other ballots were these done to?
But these were absolutely votes for Trump, but they were X. They should have had an Original Card, potentially as ambiguous, but they didn’t. Okay? And they were flagged as an undervote. Here’s an example that someone put a period and an X through it. These were flagged, originally, for Trump. And then later, they become an undervote, but they were never flagged. How did they suddenly show up as a undervote later on, even though they were never flagged for adjudication?
Another example here, another example here, and another here. So, those were the 5 that came from here to here; so, that we were able to understand why. But again, this was a lot of detailed analysis. Then, one of Biden’s pure votes, I call this the O-ring situation. Okay? So, here’s a Biden pure vote. Okay? It was a pure IsVote for him. Nothing was flagged in the CVR and it gets given to Trump. Okay?
So again, what you see here is it’s a vote for Biden here, or with an X and this then goes to Trump because someone checked off REP. But again, it was never flagged. Now, again, the officials will tell you it’s about voter intent, but the AI never flagged this. But yet, it shows up as a Modified Card. And the rule is there should be an Original Card that’s flagged – then and only then, there should be a Modified Card.
That’s not the case. That rule has been violated here and it could open up for people to create Modified Cards as they wish. The next example here is 5 of pure Biden votes, with again, nothing flagged, just like Trump’s, were also then Modified Cards. Okay? And here are those examples, Biden with an X in it. You know, this is like Biden with a scratch in it; they become an undervote. Here, it’s sort of not fully filled in. Again, how is this happening? What are the criteria? Okay? And same here. And you can see here. All right.
So, next, once we discovered this, we were able to see why these Modified Cards existed. This explains 1,180 modified undervotes. Right? But where are the Modified Cards for the 6,835 EVB Original Cards that were flagged? So, there’s still 6,835 missing that should be here.
And per policy, there should be at least 6,835 Modified Cards for the EVBs, which are flagged as undervotes. Okay? As undervotes – and these cards are missing. Did these 6,835 go through adjudication? If not, why? Okay. That’s a very, very important question.
So, in conclusion, the policy question remains is why are modified cards generated in some cases and not in others. What is the policy? So, that’s what I want to leave you with. Okay?
PHASE 1I – PART 4 – Summary
So, you’re supposed to have Modified Cards being generated, in the condition that for an early voting ballot, that it’s flagged. So, 6,835, missing Modified Cards. And then you have 11 Modified Cards showing up here, but they don’t – they were never flagged. Okay? Again, from a vote count perspective, it may have not made a difference, or are these opportunities for leaks in the system? That is what we believe because there’s the – the policy should be followed, you know, all the time without any issues.
And I say this look, from an engineering systems perspective, there’s a guy called Alan McDonald, who said, you know what, I’m not going to sign off on the Shuttle because of the O-rings. And everyone attacked him. He was vilified. It was a very small little rubber piece, which connected the two solid rocket boosters, but the shuttle blew up. So, these 11 ballots that we’re talking about and these inconsistencies are places where leaks can take place. And that’s what we’re identifying. These should be air-tight. In a system, there should be no room for any of this.
Okay, so our questions are – there needs to be full disclosure describing under what conditions a Dominion AI system decides what is a vote, write-in, undervote, overvote, ambiguous?
Is a Modified Card generated for all EVBs whose Original Cards are flagged for adjudication? That is stated policy; however, it is not being followed. Why?
More generally, in some cases, Modified Cards are being generated as they should, and in other cases, they are not, when they should.
How can a pure IsVote suddenly become adjudicated and a Modified Card be created without an Original Card being flagged for adjudication? This last point is important because that means things could be IsVotes, and later someone can decide to make Modified Cards. And if we hadn’t done this analysis, we wouldn’t have seen this inconsistency. Okay?
All right. Let me also point out this point here, as part of this Phase II: How can a pure vote, IsVote, move from one candidate to another when it was never flagged for adjudication? We’ve talked about that.
And so, the above observation is disconcerting, as it took highly specialized experts, 3 PhDs in this case, to discover this leak in the System – a vote to be transferred from one candidate to another when no adjudication was flagged.
RESULTS – PHASE III – PART I
All right. Now I’m going to go through – so, that was the CVR analysis. Now, I’m going to go to some – now I’m going to share with you the data where EchoMail® processed the 2,083,000 ballots in 2 days. Right? Put all – figured all this out. And we’re going to share with you what our results were. So, if we had gotten those ballot images, early on, at the beginning of the audit, we would have ran them through EchoMail® and it would have said what the vote should be. And then we could have compared them to the CVR that Dominions AI said, and found the discrepancies. Okay? Everyone following me?
The Dominion AI ran through its analysis and said these are what the pre-adjudicated votes should be. Our AI ran through it. And this is, it’s, this is really – I really enjoyed this part of it. Everything I shared with you was a lot of detail but you’re getting very well educated now. So, we did an initial Ballot Image Analysis – with our initial – our AI. And we assumed when we did this that all the images were the same quality. And you’re going to see what we discovered. Okay?
So, if you look at pure votes for Trump, pure votes for Biden, pure votes for Jorgensen. When mean pure, that means only when one oval was selected, per the AI. And then we had Write-ins, Overvotes, and then Other, which is Ambiguous and Undervotes. So, these are our numbers. So, just look at what EchoMail®’s AI figured out. These were according to the CVR, Dominions numbers, in the record. And you can see, we found less votes for Trump than they did, in this first pass. We found less votes for Biden than they did. We found less votes for Jorgensen, less votes Write-in. But we had lots more Overvotes and we had lots more Other votes, that should be adjudicated. Right? So, we didn’t – our AI was more conservative from the Analysis – it didn’t allocate – it would have said there would have been all these votes for adjudication.
PHASE III – PART 1 – Summary
However, we noticed something interesting – that the ballot images’ quality, when we did this first pass, was not homogeneous.
The quality of the Ballot Images generated at those MCTech, centralized 33 tabulators, were significantly better than the Ballot Images generated at the 346 in-person tabulators. And you can see these differences. This is a Ballot Image from MCTech, the centralized servers. Okay? The centralized tabulators – those 33 – where most of the early voting ballots came in. These were, about 165,000 – tabulators, which were spread out over 346 in-person tabulators – these were so light. And so, we had to do image enhancement. But when we ran it through all of them, we weren’t able to read these. So, we had to enhance because the ballots weren’t homogeneous – the images.
What we found, in fact, only 19 of the ones that came from the in-person, these guys, were even readable – which is only .02%. The rest of them were unreadable. So, to what I’m – In summary, what we’re saying is that the Ballot Images that were produced by the in-person voting tabulators, were, except for .02%, were unreadable – meaning they were different quality images.
So, we apply an enhanced Ballot Image analysis method to reprocess all those Ballot Images. If not, we would have been done, in fact, sooner.
RESULTS – PHASE III – PART 2
So, what we did was we applied – We took these Ballot Images and our system detected those that were clean, very beautiful resolution. These were called Group I – and we said, how many of those were for Trump, Biden, Jorgensen, Write-in, Undervote, Overvote, Ambiguous. And that’s this first row, which says GI.
Then we took all the ones that were misaligned among this Group. We found some of them were misaligned. We applied a technique called homography. And that was Group II. And that was Trump, Biden, Jorgensen, Write-in, Undervote, Overvote, Ambiguous. That’s GII here.
Okay? And Group III were all of those Ballot Images that came from the in-person – that were completely different. And then these also got allocated, as you can see here, by our AI. Hey, John, I need my charger plugged in immediately. Sorry. Hold on, everyone – I don’t want to lose juice here. Thank you. Okay, so we’re going to plug in because I’m running out of juice. There we go. Okay. So, what we saw again, this is the enhanced ballot – So, you can see, we have three different types of Ballot Images. This row here is the total that we got in each case. Again, everything adds up, 2,089,563. “Pad” here was what Dominion got.
And you can see the differences here. Let me go here, and you’ll see this here. Okay. So, with the enhanced Ballot Image Analysis, because we had to take into account the very hard to read ballots, you notice that this is what we get. So, this is what our AI calculated. This is what Dominion’s AI calculated. And you can see the differences here are now 2,601. They gave 2,601 more to Trump. They give 8,344 more to Biden, 102 more to Jorgensen, 7 more in Write-ins. We found 7,805 more Undervotes, 1,046 more Overvotes, 2,203 more Ambiguous votes. Okay? So, these are the differences.
Now, what we did was we said, Okay, this is where it gets interesting. So, imagine now saying, okay, in the audit our AI, this is how the election should have been. This is what our Dominion did. What about if we said, okay, where do we agree? Because if we both agree, that means we don’t really have to really worry about those Ballot Images. And the ones we don’t agree with, those are the ones we know there are discrepancies. Now you’ve reduced the problem – the audit problem – not from looking at 2 million Ballot Images, down to a finite set. And this is why I say, as you’re going to see these results, why it was, frankly, reprehensible that we weren’t given the Ballot Images and the Ballot Image stuff wasn’t done up front. And you’re going to see these numbers.
So, what do we see here? We see the common. So, out of the Trump that we figured out and the Trump that Dominion figured out, 990,011 were common. But there were 1,361 which we had a difference. And 3,962, which they had a difference. Okay.
But Biden, same thing. The common were 1,029,316 and there’s only 661 that we had a difference.
Same with Jorgensen, only 47 difference.
The Flagged for Adjudication, there were 13,000. We had 13,000 difference in the ones which should be adjudicated. But overall, I mean, they both add up because it’s accounting here, but 15,546 ballots is what would have been different in the pre-adjudicated state – so, 15,546 ballots. So, if the Ballot Image Analysis was done on day one of the Maricopa Audit, instead of having to review all those 2 million. I mean, not to say you don’t want to do that, but imagine the amount of time you could have saved because you could have found – you could have focused on these discrepancies.
I hope everyone’s understanding what I’m saying. John, is everyone getting that, in the comments? So, does everyone understand what I’m saying? If the ballot image analysis was done upfront, we would have been able to localize the discrepancies to 15,546 ballots – which is about – what percentage is that – 1% would have been about 20,000. Right? This would have been about .7% – a handful of ballots – which we could have done in weeks and allowed the audit to focus on much bigger issues.
But if you notice, what happened was, in the news, and the – it was paper ballots, paper ballots – teams of people doing this. Meanwhile, there were things in the audit that occurred, before even the paper ballots came in, the chain of custody, the signature verification. And the grifters who jumped on this, didn’t even focus on that. In fact, a lot of people got paid a lot of money to grift and the chain of custody, the signature verification, where you really needed time, was never addressed. So, what you see from here was that we could have done this Audit in a very fast timeframe by focusing on the 15,546. These are the ones in the pre-adjudicated state.
Okay now, just to give you a review here. If you look at the 25,697, which Dominion flagged for adjudications, EchoMail® flagged 36,744. So, when it comes to adjudications, we aren’t, you know – there’s agreement here. We could have looked at these, where we disagreed with, and these. Again, it’s about 15,000 ballots.
PHASE III – PART 2 – Summary
So, the summary of this is the following. The Ballot Image analysis, AI, and the Dominion Ballot Image Analysis should have, you know, concurred on 2,074,017 ballots, and don’t concur on these.
To resolve the 15,546 difference, it is necessary to know what policy – rules and standards – Dominion used in defining a vote, write-in, undervote, as we’ve mentioned. What I, the reason I say that is, suppose there are standards which say, when the circles filled in like this, and this. Well, we could program our AI to follow those rules. So, start thinking about the modern world as machines and machines, humans and humans. Right?
So, the machine, the AI machine of Dominion, analyzed the votes, but they’re not telling us what their standards were. Well, if they publish those standards, anyone listening, if you’re a programmer, should be able to write your own AI – follow those standards. And guess what, you should get the exact same results. Okay? These results – there should be, you know, zeros in these. Okay, but we don’t know them. So, that’s why we’re saying to the legislators and people listening, this is a big issue. We don’t know what the standards are.
So, did Dominion use the same – another big issue that comes out for us. And this is a significant one. We were given Ballot Images to use to do our Analysis, but did Dominion use those Ballot Images? Or did they use Ballot Images that were generated at the time of the scan, in memory? Maybe those were much higher resolution images because we find it interesting that the paper ballot scanned ones, or the Ballot Images that came from those in-person, are so horrible. We had to do a lot more image enhancement versus the other ones. So, one of the questions we want to raise: Did Dominion use those SAME Ballot Images? Or are they getting access to a version of the Ballot Images, which are much higher resolution? If that is the case, that is also a legislature issue. The real Ballot Images that they use for scanning should be what are archived and saved. Okay. So, that’s what the question here is.
And finally, is there a difference between the Ballot Images analyzed by Dominion versus the Ballot Images later archived and provided to EchoMail®? So, again, we’ve put this into a repository. And this too, is going to be given to the Senate and to the Attorney General. Okay?
RESULTS – PHASE IV
And then finally, in Phase IV, what we did was – we did something interesting. We compared our AI results to the final CANVASS Reports to see how different they were. Okay, and this is what we see here. So, here we see, here’s ours. Here’s what was finally tabulated. This is common. And again, this is a little bit – 16,437. So, that means if we want to look at the pre-adjudicated state, in an audit, we could have looked at that 15,000. If we want to look at the post-adjudicated state, we could have at the 16,000.
But regardless of whether it’s 15 or 16, an audit could have been done faster and cheaper, and in my view, millions and millions of dollars in taxpayer dollars could have been saved. So, it begs the question: Did people not want to do the Ballot Image Analysis because they wanted to grift on the paper audit, and not address the real issues and make millions of dollars? I have to ask that question. It’s not an accusation. It is a fundamental question because if we could have compressed the time for the paper audit and spent more time on the issues of chain of custody, on the issues of signature verification – by the way, which we ourselves have done on our own time. And we’re going to share with you those results. And those results are a whole other aspect that we’ve discovered and we’ll talk more about that.
CONCLUSIONS
But in closing, as I wrap this up, here are the conclusions. Again, why was analysis Ballot Image and CVR not performed as first step in the Maricopa Audit? Millions of taxpayer dollars and time could have been saved, and such effort could have been directed to real issues – chain of custody, signature verification to advance Election Systems Integrity. And as we shared, you know, the EchoMail® Ballot Image Analysis identified 15,546 and 16,437 ballots that did not concur with Dominions AI’s pre-adjudicated tabulation and the post-adjudicated tabulation, respectively. The audit should have focused on these paper ballots versus reviewing all the ballots.
Next is: Why are these 6,835 Modified Cards missing? I think we’ve talked about that. Okay?
Next is: When is a vote a vote given the inconsistent use of the IsVote flag by the Dominion system?
Next thing is: Why are any ballots, even one, being adjudicated for the Presidential Race when the Original Card was never flagged for adjudication? And we have 11 examples of those.
Another one is – One important question that remains is: Are the Ballot Images for analysis during election by Dominion AI different than the Ballot Images archived and provided to EchoMail®? This is a major question. Did we get, did we get sort of, shittier versions, excuse my language, of the images? And did they get much more pristine versions that they used? Okay?
Then finally, you know, we need to understand that the legislature, that the legislature, not a private corporation, must define the actual parameters that the AI should adhere to in determining a vote, write-in, undervote, overvote, or ambiguous. I can’t overemphasize this. The biggest conclusion, in addition to all the other things, is who is telling a private company, an AI, how to decide, what’s a vote, what’s an undervote? It’s doing it on its own. Where are the policies?
Again, in the old days, if a human being was doing it, they had a policy manual. You know, if it was this much vote, if it was the oval was filled in like this or astray – very clear. The machine is making these very, very complicated decisions. And what is – who is guiding those decisions? Right now, it appears that a few individuals may know about it, and if they do, then please publish it. Don’t attack us and say, oh, it’s published over here. Where is it?
It should be published so the actual citizens can also know, when they go into vote – I better fill it in all the way – if I fill it in and I put an X here, you know, it may not get counted or it could be an undervote or an overvote, etc. Right? Because if you fill in that and this, and you fill it in the wrong way, your vote may not get counted. So, even for the practicality of disclosure, it should be known. But one of the big policy issues that come out is the legislature must enact legislation, immediately, that must tell these companies that this is what a vote is, this is what a write-in is, this is what an undervote is, this is what an overvote is, or this is what ambiguous is.
FUTURE RESEARCH
Our Future Research. Now again, as I mentioned, had EchoMail® Ballot Images Analysis been done first – and I think I mentioned this at least 20 times, but I can’t overemphasize, and I’ll keep repeating it. And everyone should – this is one of the key takeaways – that that would have provided more time, effort and resources into the real issues.
Now as a follow up to this work and our previous EVB Envelopes Signature detection analysis, which we’re completing, we’re going to also be completing an EVB Envelopes Signature Verification analysis to determine how many EVB envelopes had non-matching signatures that did not go through the “curing” process. And this data is coming out shortly. Be ready for it. But it’s important because – by the way, EchoMail® was brought in way towards the end of the Audit.
I have no idea why the Cyber Ninja’s guys didn’t bring us in initially. We did some of the earliest analysis for this. Yet, they brought in people who didn’t have the decency to give us the Ballot Images though they had it in their possession, in uncorrupted format. And everyone should be angry with this because a lot of resources went there. A lot of grifters made money talking about all sorts of stuff. But these fundamental, important detail Engineering Systems issues were never addressed. And we’re doing it after the Audit.
That’s why our title says Why NOW. We’re doing it now because we only got the Ballot Images in December, after the Audit was done. And, in fact, at that, you know, President Donald J. Trump, or the former president, when I met with him on November 23rd, didn’t even know what Ballot Images were. So, think about that. A guy who was the potential victim of potential election integrity issues, didn’t know one of the most fundamental things. No one even advised him. Why? In my own election, I spoke about this.
This was the basis of my lawsuit with all these lawyers running around, with all these people who did the “ Audit.” Why was not Ballot Images brought to the forefront? Okay? And why is it that we have to beg to even be paid for this work? Three PhDs – I can’t tell you what my billing hours are, but they’re far more than $50,000, and we have yet to be paid for this. And by the way, people who wanted to pay for this, wanted to do this to try to gain credibility from the grifters that they were supporting, which and whose money we do not want to accept. Okay, so there you go. EchoMail® Audit of Ballot Images and Cast Vote Records from 2020 US Presidential Race.
PUBLIC CALL-INS
Hey, John, I’m going to take some phone calls, if you don’t mind. I’ll do right on my phone. Okay? So, if anyone wants to make a call, if you’re interested, and ask me a question. I’m going to take about five phone calls, John, five phone calls. And let me give you my number, 617-631-6874. That’s my personal cell phone number. And I’ll put your phone up here. Typically, we have a call-in system, but 617. John, can you scroll it on the bottom, 617-631-6874. Any questions you have on what I just covered, please feel free to call. Again, this is Dr.SHIVA Ayyadurai, 617-631-6874.
Feel free to call and we’ll go over any of this material. But in closing, the key takeaway, as I shared here, is that – Oops, I’ve got someone calling here. Let me pick up here. Hello, Dr.SHIVA speaking. Oops. Hello? Can you, can you – someone just called, but they had their phone on. Let’s see who that is. Okay, so again, if you have a question. John, keep the number up. Okay. Oh, I see. I think people are – Hello? Someone called and just hung up. Okay. So, I think what’s – Oh, my ringer was off. Sorry about that. So, if people want to call, please call, but if not, you can go to the – we have – Hello, Dr. SHIVA speaking.
Caller 1: Hi. I was just curious. I kind of missed the beginning of the broadcast. But I was wondering, did you happen to talk about like the kinematic artifacts that people were talking about at the beginning of the Audit?
Dr.SHIVA: Yeah, it’s a bunch of garbage. Okay, it’s a grifter. And it’s absolute garbage. And I think you should go explore that. I did a video on this. And it’s – there are people out there who’ve been grifting on this nonsense to distract the dedicated people from real stuff. Oh, it’s absolutely just trash, garbage.
Caller 1: Yeah, and I saw some of the previous, I don’t know, books that the person had –
Dr.SHIVA: Yeah, it’s all it’s just garbage. I did a video on this. Okay? It’s unfortunate because everyone is getting sucked in by this guy. His actual name is Jeffrey Philyaw. Okay? Go look at his name. And he has no expertise to even address this, but he’s, you know, people have been giving him money. And people should understand that this kind of stuff takes serious engineering – a lot of real hard work. And people have been, frankly, bullshitting people.
Caller 1: Yeah, I fell for it a while ago. My discernment and ability to see through a lot of this stuff has come a long way.
Dr.SHIVA: Yeah. So, this is why the people who are deniers – the people who are grifters, in my view are actually enabling the deniers. They’re spreading disinformation. It really makes you wonder whether it’s nefarious because there are real Elections Systems Integrity issues, like the ones we talked about here. Okay? There are real issues with chain of custody; there are real issues with signature verification. And the grifters and the politicians who are using those grifters, even on our stuff, have just made money off of it. Trump, frankly, raised a half a billion dollars. Where did that money go?
Caller 1: Exactly.
Dr.SHIVA: So, we need to understand there are the real people doing real work like we do, and not getting paid. Okay? And the grifters who’ve been, you know, whining about not being paid, but they’ve made millions of dollars.
Caller 1: Yeah, exactly. Exactly.
Dr.SHIVA: So, it’s time that people call these people out. Cyber Ninjas had these Ballot Images; they sent us corrupted stuff and then they blamed the County. So, of course the County is going to get upset with them. You see what I’m saying?
Caller 1: Exactly.
Dr.SHIVA: So, people need to wake up, that this is not left or right anymore. So, the establishment wants to make this a left or right issue. So, we don’t, so of all the real issues are put under the rug.
Caller 1: Exactly.
Dr.SHIVA: There are serious issues with chain of custody; there are serious issues with signature verification; there is a serious policy issue here; you have AI technologies which are determining these things. And the legislators. I mean, they have all these bills there – this should be a bill that should be in there – in every legislature in the United States.
Caller 1: Exactly.
Dr.SHIVA: Okay.
Caller. 1: Thank you.
Dr.SHIVA: But I appreciate your calling in and everyone needs, like you just said, to raise their discernment levels. Stop following these grifters. They’re scumbags. They really, really –
Caller 1: You can’t believe it anybody but yourself. Yeah.
Dr.SHIVA: Yeah. Look, I can’t tell you – you guys know I try to do videos – I haven’t done a video in, for a while. I literally, and with my other two associates – I mean, this has been hard work. You cannot do this grifting. That 1000-page report that that fool did – that is what he is. Okay? – is just garbage. It’s end-to-end rubbish.
Caller 1: Damn right. Thank you so much, Dr.SHIVA.
Dr.SHIVA: Yep. You’re welcome. Hello, Dr. Shiva speaking. Hello. Hello, do you have a question?
Caller 2: I do.
Dr.SHIVA: Sure. Go ahead.
Caller 2: Yeah, it’s about, obviously, Jeffrey Foolya.
Dr.SHIVA: Yeah, yeah. What about him?
Caller 2: Well, I just want to understand, you know, why finding fake ballots is not of any importance.
Dr.SHIVA: Why finding fake ballots? What do you mean?
Caller 2: Correct. And he’s not the only one. There was also another one, and I can’t think of his name, that’s also confirmed that that has happened. So, while I get what Shiva was saying about people making money and all that other stuff –
Dr.SHIVA: Yeah, well, here’s the deal. Do you know what evidence is? Evidence? Evidence is actual evidence. Okay? So, you go through this – you go through his report. You should go do, see a video I did. The guy has not produced any evidence, at all. And he’s actually hurting –
Caller 2: I’ve got, you know, I’ve looked at some of the reports he’s put out. And there’s another gentleman, I can’t think of the name of, who’s also a document examiner, who’s also confirmed that they found fake ballots. And –
Dr.SHIVA: Well, let’s, let’s – you should send them to me. Okay? Because everything I’ve seen out of his stuff is just rubbish.
Caller 2: Well, I mean, I don’t see like, the front and the backs of ballots not lining up and the out of alignment and the types of paper that were used –
Dr.SHIVA: Yeah. So, go through that, go through that report carefully. Okay? And you’ll find out the pictures that he’s showing there are not from ballot paper. Okay? My point is – And you should go through it very carefully. Because there’s the real Election Integrity Movement and there’s the fake one. And there are people who’ve been running the fake one. So, you need to have –
Caller 2: I don’t doubt that there’s, you know, multiple factions here working against each other. But I mean, from what I’ve seen, and I’ve got to find this guy’s name, but you know, the other guy that, that also, you know, came in and looked at the actual paper and confirmed that there was fake –
Dr.SHIVA: Yeah, but what I’m saying is, you know, people confirming – I think you should really, really look at this closely. Okay?
Caller 2: Oh, I. have. I follow this extremely closely. I mean, I watched everybody. I don’t, I don’t trust anyone.
Dr.SHIVA: So, what I’m saying is, you should go actually, yeah, you should actually go study the details of that. Okay?
Caller 2: I have. I mean, I’ve looked at it quite thoroughly. I think that there’s a lot to it, including the, you know, the fake ballots. You know, I think that they were, you know, the adjudication and the inflation of the voter rolls, you know, it all plays together. But I think that they ran out of official ballots, at some point, and they had to pump in some fake ballots. You know –
Dr.SHIVA: Yeah, but the thing –
Caller 2: Here we’re trying to confirm that with the ballot envelopes, you know, you’ve got ballot envelopes that were clearly scanned in multiple times, and then doctored between the times they were scanned in. So, you got the exact same –
Dr.SHIVA: Well, let me – wait, let me be let me be very – we have to take a Scientific Systems Approach. Okay? What we discovered – you know, we, when we presented our stuff on the envelope images, we made very clear that these were anomalies. Okay? And what came out of that was the understanding that every time an envelope image comes in. Okay? If that envelope’s image – So, let’s say 2 million envelope images, and we’ll do another assessment on this – when 2 million envelopes – See there’s a lot of people don’t even understand this process.
The gentleman you’re talking about didn’t even understand what adjudication was. It’s – these are not easy concepts. The stuff I went through today, I would say 99.9999% of the public doesn’t even know- it has never been explained to them – but when you take the process of when ballots come in and envelopes, first process that happens is they’re all scanned – the envelopes. Then, people are supposed to review them within 4 to 30 seconds. All right? And decide if the signatures match. If the signatures do not match then what happens is they are sent for curing. In Maricopa, less than 1% were cured, about 25,000. And then of those that were cured, 587 were found to have signature mis-matches – which is point .03% – which is three one hundredths of a percent. This is a big issue. Okay?
Caller 2: I wasn’t really talking about, you know, ballot curing. I’m talking about you’ve get three separate images of what is supposed to be three separate ballot envelopes. But two of the images – I mean, all three of them bear being exact same handwritten date, but two of them have a stamp placed on them and one of them doesn’t. So, it was scanned in –
Dr.SHIVA: Yeah. Listen, anything coming out of that – anything – Yeah, let me let me just finish this. Anything coming out of that guy is suspect? So, I have all the – yeah, but –
Caller 2: But your findings –
Dr.SHIVA: No, no, no, no, what I’m saying is – There’s two things here. You have to understand the findings here. Okay? When we did that analysis, the main thing we wanted to share was there were these anomalies. Okay? The result of that was to show that the envelopes were being cured, and that’s when “ duplicate images” were being created. Do you understand that process?
Caller 2: Well, when you’re saying, cure, does it not have to be cured by the voter themselves? So, when
Dr.SHIVA: No, no, no, no, no, no, no, no, you’re not understanding the process. Okay? So, this is what happens. When all the images go in, they go to an organization called Runbeck. Okay? That organization then, human beings, reviews every envelope image. Is that clear?
Caller 2: Yep.
Dr.SHIVA: Okay, so on one side, they have the original envelope image. On another side, to the right, they have the signature of that person from the voter registration record. So, if some guy’s name is John Doe, John Doe signs and they have John Doe signature. Within 4 to 30 seconds, these volunteers have to review that and decide if it is a bona fide signature. Got it? That’s step one. Is that clear?
Caller 2: Yeah.
Dr.SHIVA: If they match, then the envelope is opened and it’s processed. If they do not match, okay, if they do not match, then it’s sent for curing. Curing means that goes to other sets of human beings who call the person up and decide if that person is a person who they say they are. Got it?
Caller 2: But how does it end up scanned into the system multiple times?
Dr.SHIVA: That’s what I’m trying to tell you. This is what happens. So, what – in the curing process, if something doesn’t match, they take the original image and then they scan it again, and then they put the stamp verified and approved. Okay? That’s part of the curing process. So, that means the images that we found that were duplicates were the ones that were cured – which is 17,000, like 200 images – 1,333. Okay. So, the duplicates are the ones that were cured. The more important issue is, why we’re only 17,000 cured? Do you understand what I’m saying?
Caller 2: Well, shouldn’t there be more ballot envelope images than ballots? If that’s the case, that they’re scanning the ballot envelope images in multiple times?
Dr.SHIVA: There is. There is more ballot envelopes images than – there is. That is a fact. Anyway, watch for – watch for my broadcast two days from now. Okay? We’re going to discuss all this. But appreciate your call. Thank you. All right. So, let’s take one more call. So, that was a question on – someone else called here. Your call has been forwarded to an automatic voice. It is – Here we go. Hello, Dr.SHIVA speaking.
Caller 3: Dr.SHIVA, hi. I am a cybersecurity professional, taking with interest, in the Arizona part of it and –
Dr.SHIVA: So, do you have a question on the on the Ballot Images’ stuff we just covered?
Caller 3: Yes. I mean you’re showing great science, the scientific perspective of this, and I’m totally in-sync. But what I’m also seeing is, hitting it from all different perspectives, right? I understand your point of view about people making things up or giving you garbage – you know, maybe the accusations of, you know, chain of custody, Senator Rogers, Mike Lindell with the China hackings, ballot harvesting accusations and from my perspective, I’m concerned about the hardware itself, as well. There’s discussions and even documentaries, that are dating back as far back to the 1980s, of actual chips in these voting systems that could have been maliciously changed or updated without people knowing. My cybersecurity experience – you know, it’s one thing to get the scientific aspect of it, but when you’ve got multiple vectors of attack, how does your approach – might help knock those trees down, per se? Because you know, you might have Maricopa County say one thing, you know, which is your evidence, but at the same time, we might also have sordid other things that are happening as well, in other counties and that are related. I tend to find –
Dr.SHIVA: Your voice is going in and out. Can you speak closer? Your voice is going in and out.
Caller 3: Oh, okay. Sorry. All right. Well, I tend to find as a security cybersecurity professional that when you start finding problems, you start looking more. And sometimes the obvious is the obvious, sometimes it’s not. And you just keep probing until you stop finding problems. So, I guess my question to you is, aside from your audit, what else can be done, at this point, when we might have multiple attack vectors in place?
Dr.SHIVA: Well, yeah, so you’re asking a good question. Look, the reality is, if you look at this as, first of all, from an Engineering Systems perspective and you look at the entire – you have to look at the whole process. Okay? End-to-end. You can’t just look at one little thing. That’s a very reductionist way and you can manipulate people. That’s what the grifters have been doing in this movement. Okay? They’ve been just taking advantage of people, not talking about from an engineering – and many of them don’t even have an Engineering Systems Approach to this. They have a grifter engineering approach. Okay? Grift on something. Okay?
So, I say this with some level of facetiousness but more some serious concern. Because when you look at the entire end-to-end process, there are some big elephants in the room that have not been discussed. And these are things that you don’t even have to say, oh, did China do it. Did this happen? You know, I love Mike. Right? I went to his cyber-symposium. And if you remember the numbers he put up, it was sort of, frankly, ridiculous because if you looked at the numbers, it was all about China, China, China, showing these things coming from elsewhere. If you remember that, right? The problem was when you looked at the final numbers he displayed, of what the votes were for Trump, with what they originally, what they were reported – if you looked at those votes and you put them in a spreadsheet – and I was there and I said what the hell is this – if you divided the votes that the claim was with, you know, what they were hacked by. Right? That was all exactly 4.2%. I don’t know if you remember, I did this thing on the back of an envelope right there, on a quick spreadsheet.
Caller 3: I did, when I was watching this in front of me –
Dr.SHIVA: Yes.
Caller 3: – as a CISSP, you would want to see the data.
Dr.SHIVA: Yeah, but what I’m trying to say is when Mike finally said okay, I’m going to now reveal the numbers – how many votes that Trump should have gotten and what it was. Right? If you divided what he got from what Mike reported that the hacker said he actually got. Right? In all 50 states, it was plus or minus .1%. 4.2%, okay? There’s no way hackers just multiply votes by 4.2%. Okay? So, I, frankly, have lost my patience on this because I know what it takes to get your hands dirty and go in there and look at this stuff. It is not easy. And there are real issues here. And, you know, talking about China, China, China, when you don’t really have the evidence for it, is in some ways obviating or misdirecting the issue from people right here doing it. Okay? Whatever that is.
Caller 3: Just to interject here and this might not be specific –
Dr.SHIVA: But no, no, I just, I just, but do you understand what I just said? That the so-called China thing that was hacked was exa –
Caller 3: – was readily available when he was. I know he’s a salesman. showman, just like Trump is. I like Trump as well. But, you know, he didn’t show the hard data on the website and is still not showing the hard data. I tried converting some of the Excel data that he converted to, you know, the webpage. But then I start looking at it, and I’m like, I still need to see the packets. And everybody’s complaining, where are the packets?
Dr.SHIVA: I know. That’s what I’m trying to say. I’m trying to say we’re in an era – there are three groups in any problem = the people want to deny the problem, the people who take a real problem and grift off of it, and those people are in some ways worse than the people who deny it, and then there are people will actually have to spend a lot of time in finding out, teasing out the issues. Engineering and science and, you know, finding stuff, is not easy. Grifting is a lot easier. So, we have to call out these grifters hard because what’s going to happen is the grifters are actually supporting those in the establishment who want this issue to go away.
Because they’re making outlandish, ridiculous bullshit that is almost like giving a layup to the opposition and I’ve seen this in many, many other areas. It’s like classic, you know, psyops’ techniques. You know? You don’t want to discuss the real issue, so you talk about something else. So, you can’t, you know – we’ve got to shut that nonsense down. And that’s why, you know, everything here is going to be up online. We’re going to ask the Maricopa officials to an open question. But everything here is completely unassailable. It’s defensible. It’s not like, you know, throwing some images in a thing and saying, you know, you found kinematic bullshit detection. It’s just garbage, just fucking garbage. Excuse my language.
Caller 3: No, you’re the most thorough that I’ve seen. You know, like you’re saying, the grifters are out there. I mean, I don’t know if Mike’s a grifter, but I think he’s a showman, he’s upset and trying to help –
Dr.SHIVA: Well, I don’t consider Mike – well, first of all, I don’t consider Mike – I don’t consider Mike Lindell a grifter. I think Mike is a well-meaning person. And I think he cares deeply about this country. But I think he has a judgment issue on who he gets information from. Okay?
Caller 3: Yeah, that’s what I was wondering about –
Dr.SHIVA: But there are the people that are feeding him. The people – there are other people who are actually, knowingly, doing bullshit and raising money. And those people should be absolutely defecated upon. Okay? Thank you.
Caller 3: I think you think your audience is feeling the pain too. We want results and –
Dr.SHIVA: Well, what I just showed here –
Caller 3: There is some data. You’re showing it. You have to really pay attention to understand what it is because not all of us have PhDs, but it’s sensible. It’s understandable if you just pay attention and ignore all the other traffic out there.
Dr.SHIVA: Well, you’ll see in a few days. So, this, what I’m sharing with you, is actual Ballot Images – okay – the actual CVRs, the actual minutiae of analysis, tracing this. And it’s a lot of time. And this was another PhD here in many ways. Okay?
Caller 3: Right.
Dr.SHIVA: And these people who are grifters do not value hard work. They don’t really know what real analysis is. They’ve never done it. And they think you can bullshit your way through life. And they’re actually hurting our movement. I mean to sit down and actually solve a problem is a ton of actual effort. These people have been getting away – bullshitting. I mean you’re talking about this guy Philyaw who said he had discovered the Ark of the Covenant. Okay? He’s involved in Election Integrity? Come on. You can’t have people who have no integrity involved in Election Integrity. So, we’ve got to wake the f’ up. Okay? Thank you.
Caller 3: Absolutely.
Dr.SHIVA: All right.
Caller 3: I am going to go ahead now and get off the phone and –
Dr.SHIVA: Okay. Very good questions. Oh, no, but you’re right. It’s –
Caller 3: I appreciate it and thank you very much for your hard work.
Dr.SHIVA: Yeah. Thank you. You’re welcome. All right. We’ll take one more call. John. Any questions people have?
John: Oh, people were asking about, you know, how can you trust that these are the actual Ballot Images.
Dr.SHIVA: Okay, so yeah, so we have a call from Michigan. This is our last call for the evening. How are you?
Caller 4: Hi, I’m good. My name is Andrew.
Dr.SHIVA: Hey, Andrew, how are you? I’m good.
Caller 4: I’m good.
Dr.SHIVA: What’s your question, Andrew?
Caller 4: I wanted to ask about Dr.SHIVA’s implementation, his opinion, on how to implement legislation for AI Systems?
Dr.SHIVA: Yeah, you’re asking a great question. Yeah, so –
Caller 4: Well, I can’t hear Dr. Shiva. Sorry, I can’t hear him, but –
Dr.SHIVA: No, this is Dr.SHIVA. Go ahead.
Caller 4: Oh, okay. Sorry, I’m suffering a lag time. What I wanted to ask you was when you talk about legislation – now AI is rather a black box. It’s something, you know, where you have to train it in order to do a certain function. And my question for you was considering how this is kind of, you know, as you talk about in your courses, with a system, with feedback, and noise and interference. Do you think that an AI system is something that a legislature could adequately create, you know, guidelines for how to train it? What is an acceptable mark and so forth? I’m just curious of what your opinion is on that.
Dr.SHIVA: Yeah, you’re asking – yeah. Look, I mean, we’re heading into a virgin territory now. Right?
Caller 4: Yeah.
Dr.SHIVA: The reality is – and this is why the problem with legislators – I mean, by the way, I don’t know if you know this? In China, for example, most of the members of the Communist Party are like engineers and scientists. You know? Most of the legislators in the United States are – I don’t know what the hell they are. Okay? They’re lawyers, on a good day. So, the problem is the legislators in the United States, in the modern world, are always 20 years behind technology. This was not the case. This was not the case at the time of the Founding Fathers. You know, Franklin was an inventor. Okay? Washington was a farmer. Right? And a businessman. Right? He was a surveyor too. Jefferson was an architect.
These guys had the vision to create the modern patent system. Think about that. They knew what intellectual property would mean because they themselves were creators. They valued it. Okay? The reason the United States did so well was because of the IP protection laws that were created. You know, at MIT, for example – it created 33,000 companies, which today add 1 trillion to the GDP, because of the IP protection laws. That came from the Founders. Now you have a bunch of dumb fucks who are, excuse my language, who are in politics. Okay? They don’t even know what AI means.
Give you my own personal example. In 1978, when I created the first Email System, which I did – and when I mean Email, I’m talking about the System, which was the emulation of the Interoffice Mail System, inbox, outbox, all those things – I did it as a 14-year-old kid in Newark, New Jersey, before I came to MIT – in an environment where no innovation is supposed to come out of, but I did it as a 14-year-old. So, when I came to MIT, it was featured on the front page. The president of MIT, Paul Gray, he said, you know, Shiva, you should copyright it. This is a 1981. He had known that the laws, you couldn’t patent software in 1981, even, because the Supreme Court wasn’t recognizing patents. This is how stupid politicians are.
It took until 1994 for them to realize software is a digital machine that you should be allowed software patents. So, I wasn’t able to patent it, but I did copyright it. By the Copyright Act of 1976, sorry, by 1980, software could be protected by copyright law. Okay, so I did it. So, I got the – I’m recognized, I named it email, called it, etc. 35 Five years later, 36 years later, my stuff went into the Smithsonian. It created this “controversy” created by the elites who could not imagine that a 14-year-old kid in Newark could invent anything. You see what I’m saying? Now the reason I bring that up is – so, it took the politicians 16 years. Right – 1978 to 1994, to even create the concept of software patents. So, this is why we have a problem in the United States. And it’s going to degenerate if we keep electing idiots. Because these idiots are going to hurt this country in a very profound and deep way. Just like Trump saying he didn’t know what Ballot Images were. Think about that.
Caller 4: Yeah, it was shocking to me –
Dr.SHIVA: It’s shocking. When I was with him and that was my second meeting with him. And none of his lawyers had advised him. That’s further shocking. Okay? So, the reason – and I’m always pretty much either 5 to 20 years ahead. Okay? And I can see the things because I’m on the ground making – anyone who’s actually working for a living can see the future. Anyone who is grifting can never see anything. But what I can tell you, right now, is that the Dominion AI machines are the equivalent of a bunch of humans working. Right?
They are deciding how much – I mean, their expert rules have that. What I’m saying is those rules, the decisions that those systems are making, should be printed and reviewed. You see what I’m saying? Because they are, ultimately, algorithmic. They are – whenever you used to do AI, at least, you know, you’re supposed to do what’s called backward chaining and forward chaining, to be able to see how a decision was made. We needed 3 PhDs to go through this to figure all this – what I just shared with you was a serious exercise – in finding those 11 votes – I mean, it was it was killing us – like how could this shit happen? That’s what the Audit should have done. You know, Doug Logan, who was running the Audit, was out there raising money all day. Then you had this guy, Philyaw, fooling people.
Why did you do all these paper audits all day? Why didn’t you do the Ballot Images in 4 weeks? They were grifting, man. Meanwhile, big issues got forgotten about. There were major policy issues – major compliance issues. So, we live in a world now where there are three groups. Two of them are together – the grifters take a real problem and fake it. And I, you know, I gave Trump a lot of money – supported him. But I have got to say he became a grifter, in my eyes. He took a half a billion dollars. And what did he do with it? We asked him, when I met with him, I said you know what you – we need a real Elections Systems Integrity Institute. Because the “ left,” – they have Harvard, they have Stanford, they have MIT – who just denies it. And I know my work helped them raise quite a bit of that money. They used our stuff.
And there are politicians right now running for office, grifting off election fraud, but they don’t really want to solve it. So, I hope this work that I shared today, wakes people up. I mean, this is real stuff. And it takes a lot of effort to do this. And people have to get out of the realm of these grifters. This happens in every field, even in the vax, no vax field. You know? Everyone creates a dialectic. They don’t want to go after the real Systems issues. But I appreciate your question. Thank you.
Caller 4: Yeah, if you don’t mind, I’ll just kind of summarize what I’m hearing. I think a lot of it – it’s another – You’re making another very strong point for why we need fresh blood. We can’t have stagnation in Congress and so forth.
Dr.SHIVA: Well, as long as the elections – that’s why I don’t think these guys ever want to solve any of these issues because elections are selections.
Caller 4: Right. Exactly.
Dr.SHIVA: Both parties keep it all fluid and now they have grifters running around coming up with fake issues. You know? So, we don’t address the real issues. These grifters are really bad people. Okay?
Caller 4: Yep.
Dr.SHIVA: There are substantive issues – by real dedicated people like myself and others, and you sound like one of them, right – that are not being addressed. And therefore, the grifters are playing into the hands of the establishment; they are a wing of the establishment.
Caller 4: Yeah,
Dr.SHIVA: Yep.
Caller 4: Well, I appreciate your response. And thank you for your time. Appreciate all you are doing.
Thank you.
Dr.SHIVA: Thank you. All right, everyone, it’s getting late. I’ve had probably – maybe 1 to 2 hours of sleep over the last 3 to 4 weeks. And I appreciate you guys taking time to listen. I appreciate – I hope all of you will study this video carefully. Share it. But we have got to move out of the fake nonsense of election integrity, to the real stuff. Those of you who are interested, you can go to Election Systems Integrity Institute.
We have our publishing wing which we’re creating, but we’re also going to create a wing to provide infrastructure to those of you who want to do real election work. But, please, have discernment. These grifters have got to be set aside. And we must go back to the central issue that we discussed today. There are real issues. And what we’ve discovered here, is the fact that we need immediate legislation, for the AI on these Systems – the standards that they use – to be fully disclosed and legislators telling those AI Systems how they should be functioning. Right now, private companies are doing what they want, and in many ways. And really, the standards, as we’ve shown here, are inconsistent. So, this is a real Election Systems Integrity issue. Thank you, everyone. Have a good evening.
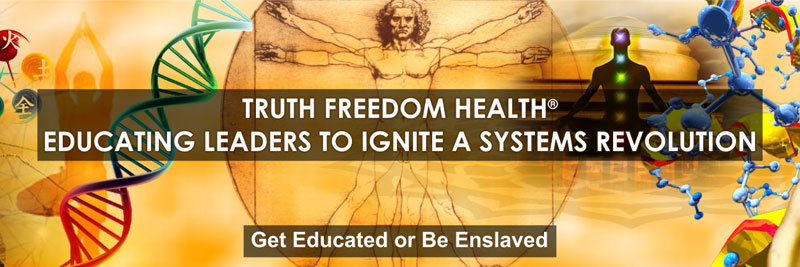
It’s time we move beyond the Left vs. Right, Republican vs. Democrat. It’s time YOU learn how to apply a systems approach to get the Truth Freedom Health you need and deserve. Become a Truth Freedom Health® Warrior.
Join the VASHIVA community – an integrated EDUCATIONAL, COMMUNICATIONS – independent of Big Tech -, and LOCAL ACTIVISM platform to empower YOU to actualize Truth Freedom Health in your local communities by employing a SYSTEMS APPROACH.
The platform we are building for Truth Freedom Health® provides the infrastructure to take on Big Tech, Big Pharma, and Big Academia. Many of you have asked how you can help. You can contribute whatever you can. Based on your level of commitment to get educated, I have also created some wonderful educational gifts to thank you for your contribution.
To get the education you need and deserve, join Dr.SHIVA on his Foundations of Systems course. This course will provide you three pillars of knowledge with the Foundation of Systems Thinking. The three pillars include: 1) The System Dynamics of Truth Freedom Health, 2) The Power of a Bottom’s Up Movement, and 3) The Not So Obvious Establishment. In this course, you will also learn fundamental principles of all systems including your body.
Course registration includes access to his LIVE Monday training, access to the Your Body, Your System tool, four (4) eBooks including the bestselling System and Revolution, access to the Systems Health portal and communications tools – independent of Big Tech – including a forum and social media for you to build community with other Truth Freedom Health Warriors.
This course is available online for you to study at your own pace.
It’s time to Get Educated, or Be Enslaved.